The Omicron Bind

The omicron variant of the SARS-COV-2 virus, widespread testing including the newly available (in US) antigen tests, or some combination of these factors has resulted in a huge number of both cases and deaths attributed to SARS-COV-2 despite widespread vaccination. Cases and deaths have soared in groups and regions such as Israel and the UK reporting very high vaccination rates, making attributing the cases to the “unvaccinated” implausible.
This has put public health authorities such as the US Centers for Disease Control and Prevention (CDC), WHO, and other agencies around the world in a bind. At best, the soaring cases and deaths reflect either extensive failure of the current vaccines or high false positive rates in the various tests resulting in other respiratory disease cases and other deaths being misidentified as COVID-19.
For the sake of argument, accept that the vaccines are quite safe despite the alarming VAERS data in the United States and that, as widely claimed, the vaccines do confer some reduction in death and severity of illness for a brief few month duration, nonetheless the huge number of cases and deaths throughout the world suggests that the vaccines are largely ineffective in real-world conditions. This may be due to omicron mutating around the vaccine induced immunity based on earlier variants of SARS-COV-2, the frequent waning of the immunity conferred by inactivated vaccines, or even other causes not identified.
This failure of course is an embarrassing debacle at best for public health authorities and agencies, political leaders, and various billionaire philanthropists, particularly because the costly and disruptive lockdowns were justified to protect the vulnerable until a life-saving vaccine would be available – despite the well known high failure rate of research and development, often estimated at 80-90 percent.
The other explanation, in full or in part, for soaring COVID cases and deaths which preserves the putative vaccine “miracle” is that the various tests for SARS-COV-2 and COVID-19 have high false positive rates, that a substantial number of the deaths are “with COVID” rather than “from COVID,” a simplistic binary interpretation of the causes of death, and other “bad counting” explanations. In the last few months, we have seen more and more publications and public announcements moving in this direction such as: https://www.reuters.com/business/healthcare-pharmaceuticals/cdc-reports-fewer-covid-19-pediatric-deaths-after-data-correction-2022-03-18/ , https://jamanetwork.com/journals/jamanetworkopen/fullarticle/2790263, and possibly https://www.clarkcountytoday.com/news/probe-finds-officials-miscalculated-covid-19-death-toll/.
However, if current omicron cases and deaths reflect high false positive rates, the past case and death counts since March of 2020, often described by public health authorities or mainstream news reports citing unnamed public health authorities when reported as both “undercounts” and highly accurate are even more suspect than recent numbers. In the United States, the CDC has previously explained the lack of real time data reporting and other flaws in the COVID-19 case and death data, causing officials to rely on data from the UK and Israel, as caused by antiquated IT systems, lack of funding in previous budgets, alleged cuts by the Trump administration, and similar excuses.
Many of the early tests were produced in haste, rushed out under experimental use authorizations (EUA), including an embarrassing failure by CDC early in the pandemic to produce a usable PCR test (URL: https://arstechnica.com/science/2020/04/cdcs-failed-coronavirus-tests-were-tainted-with-coronavirus-feds-confirm/ ). Tests, testing methods and technologies should certainly have improved in the last two years of the pandemic; if not, why not? Especially given the trillions of dollars spent on the pandemic response.
Some mainstream reporting on problems with the US CDC’s data and data handling.
https://www.politico.com/news/2021/08/15/inside-americas-covid-data-gap-502565
https://www.politico.com/news/2021/09/13/cdc-biden-health-team-vaccine-boosters-511529
https://www.politico.com/news/2021/08/25/cdc-pandemic-limited-data-breakthroughs-506823
https://www.politico.com/news/2022/03/21/cdc-email-data-walensky-00018614
https://www.theverge.com/2022/3/22/22990852/cdc-public-health-data-covid
The current crisis in the Ukraine has undoubtedly distracted much of the public from the omicron bind. Nonetheless the soaring cases and deaths attributed to the omicron and post-omicron variants of SARS-COV-2 appears to reveal gross contradictions in the claims by public health authorities about the COVID pandemic. While it is usually possible in practice to find some convoluted, acrobatic explanation for obviously contradictory data and/or logic, such explanations are rarely true.
Improper Scientific Practice
The public health authorities are portraying the flip flops and contradictions in their assertions about COVID as brilliant scientific discoveries — new science or the science has changed — although that excuse is wearing thin. This is not how proper science functions — even major breakthroughs. It proceeds from tentative statements and numbers with large error bars and/or broad confidence intervals to smaller and smaller errors as more data, better measurements, and better models are developed.
Error bars are graphical representations of the variability of data and used on graphs to indicate the error or uncertainty in a reported measurement. They give a general idea of how precise a measurement is, or conversely, how far from the reported value the true (error free) value might be. Error bars often represent one standard deviation of uncertainty, one standard error, or a particular confidence interval (e.g., a 95% interval). These quantities are not the same and so the measure selected should be stated explicitly in the graph or supporting text. Error Bars, Wikipedia, March 25, 2022
Science rarely jumps from super-confident statements such as “masks don’t work” to grossly contradictory super-confident statements such as CDC Director Robert Redfield’s ludicrous “masks will stop the pandemic in 8-12 weeks” statements in the summer of 2020. (LINK: https://people.com/health/americans-wore-masks-drive-this-epidemic-to-the-ground-says-cdc-director/ ) That sort of a jump or contradiction usually indicates bad science — gross underestimation of the errors before or after the jump (or both). In most cases, the scientific discovery is reflected in a sharp discontinuous drop in the error bars due to a better theoretical and/or mathematical model or better measurements or both.
For example, Johannes Kepler’s discovery of the elliptical orbits of the planets combined with superior measurements with the new telescopes in the 17th century resulted in a dramatic drop in the error bars on predictions of planetary motions from about a one percent (1%) error with the Ptolemaic system to a tiny fraction of one percent. It did not result in a gross reversal of centuries of astronomical observations and predictions. Ptolemy and his successors knew their model was imperfect and said so. Mars did not suddenly stop backing up for two months every two years in 1605 when Kepler realized what was going on. The empirical phenomenon did not somehow reverse overnight, rather our understanding leaped forward and the accuracy of the predictions went up dramatically.
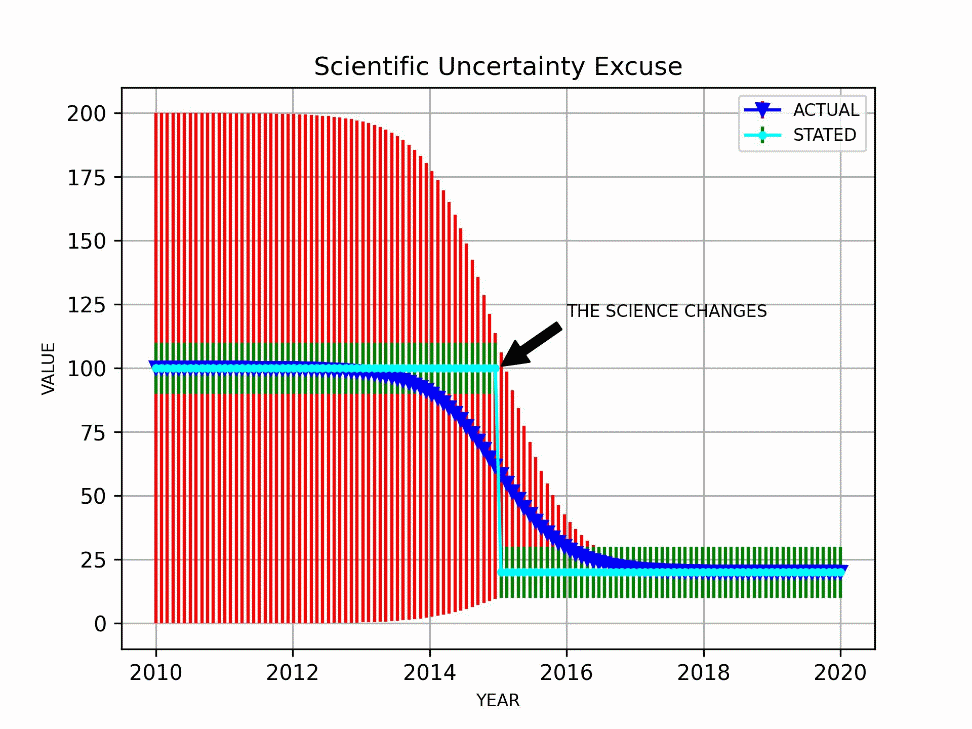
(ABOVE) The red error bars and the dark blue data points show the ideal proper scientific practice in which the reported red error bars include the actual value largely determined in the 2015-2016 period in the hypothetical example shown when the science jumps forward. The green error bars and light cyan data points show improper scientific practice in which the scientists are over-confident both before and after the “breakthrough.”
It is common for over-confident scientists to explain the contradiction by referring to the uncertainty of science as if the poorly educated audience or critic is unaware of uncertainty and as if the scientists properly reported the large pre-2015 red error bars previously whereas they actually reported the incorrect small green error bars. This switch is the scientific uncertainty excuse.
Indeed there is a frequent improper failure to report statistical and systematic errors throughout the public health “science” both as presented to the lay public, on news shows, and in CDC and other web sites and publications. One of the most striking examples is a large difference in the number of deaths attributed to “pneumonia and influenza” on the US CDC FluView website (~188,000 per year) and the US CDC leading causes of death report (~55,000 per year). These grossly contradictory numbers have been reported for years with no statistical or systematic errors, nor clear explanation for the difference. The discrepancy between the FluView website and the leading causes of death report predates the COVID-19 pandemic by several years. This gross discrepancy is likely extremely relevant to the question whether a death is “with COVID” or “from COVID” or some intermediate case.
The CDC FluView website shows that 6-10 percent of all deaths, varying seasonally, are due to (the precise language on the graphic) pneumonia and influenza (P&I) according to the vertical axis label on the FluView Pneumonia & Influenza Mortality plot. The underlying data files from the National Center for Health Statistics (NCHS) list, as mentioned, ~188,000 deaths per year attributed to pneumonia and influenza.
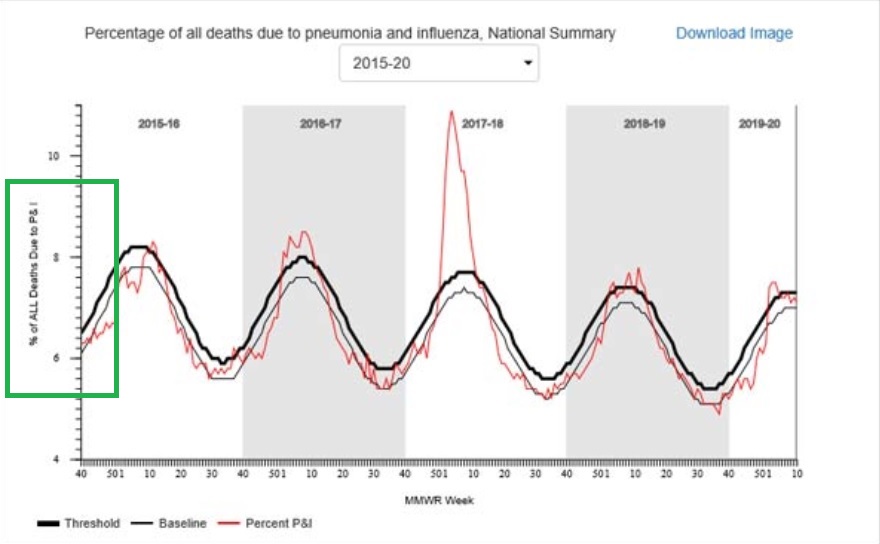
NOTE: https://www.cdc.gov/flu/weekly/fluviewinteractive.htm and click on P&I Mortality Tab
The CDC FluView graphic and underlying data files list no statistical or systematic errors. The counts of deaths in the data files give the numbers to the last significant digit, implying an error of less than one count, one death, based on common scientific and engineering practice.
In contrast, the CDC’s leading causes of death report Table C, Deaths and percentage of total deaths for the 10 leading causes of death: United States, 2016 and 2017 on Page Nine (see Figure 3) attributes only 2 percent of annual deaths (about 55,000 in 2017) to “influenza and pneumonia.”
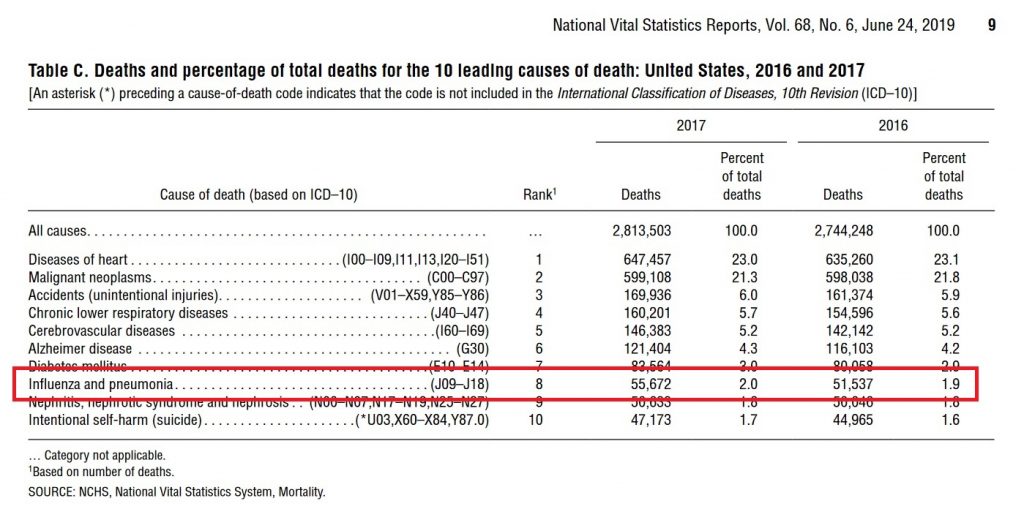
The difference between the CDC FluView and leading causes of death report numbers seems to be due to the requirement that pneumonia or influenza be listed as “the underlying cause of death” in the leading causes of death report and only “a cause of death” in the FluView data. This is not, however, clear. Many deaths have multiple “causes of death.” The assignment of an “underlying cause of death” may be quite arbitrary in some or even many cases. Despite this, none of these official numbers, either in the leading causes of death report or the FluView website, are reported with error bars or error estimates, as is the common scientific and engineering practice when numbers are uncertain. The leading causes of death report for 2017 reports exactly 55,672 deaths from “influenza and pneumonia” in 2017 with no errors– as shown in Figure 2.
It is impossible to perform an accurate cost benefit analysis of any policy without honest reporting of the uncertainties/error bars. The overconfident statements will have serious real world consequences in human lives unless they prove correct through luck.
Generally statements with — in fact — large error bars should not override personal judgment (e.g. mandates) especially in life and death situations. The government may be justified in preventing parents from treating an illness with a fatal dose of cyanide, where the lethality of the “treatment” is certain. The government is certainly not justified in compelling parents to treat an illness with an experimental treatment with large uncertainties and unknowns even if that treatment might save the child’s life.
Scientists have an ethical obligation to honestly compute and report both statistical and systematic errors; this is common scientific and engineering practice taught by accredited universities and colleges throughout the world.
(C) 2022 by John F. McGowan, Ph.D.
About Me
John F. McGowan, Ph.D. solves problems using mathematics and mathematical software, including developing gesture recognition for touch devices, video compression and speech recognition technologies. He has extensive experience developing software in C, C++, MATLAB, Python, Visual Basic and many other programming languages. He has been a Visiting Scholar at HP Labs developing computer vision algorithms and software for mobile devices. He has worked as a contractor at NASA Ames Research Center involved in the research and development of image and video processing algorithms and technology. He has published articles on the origin and evolution of life, the exploration of Mars (anticipating the discovery of methane on Mars), and cheap access to space. He has a Ph.D. in physics from the University of Illinois at Urbana-Champaign and a B.S. in physics from the California Institute of Technology (Caltech).